Abstract
- Framingham Risk Score Interpretation
- Framingham Risk Score Calculator
- Framingham Risk Score Calculator Pdf To Jpg Converter
- Framingham Risk Score Calculator Pdf To Jpg File
- Framingham Risk Score Calculator Pdf To Jpg Online
This Australian absolute cardiovascular disease risk calculator has been produced by the National Vascular Disease Prevention Alliance (NVDPA) for the information of health professionals. The calculations are based on the recommendations in the Guidelines for the assessment of absolute cardiovascular disease risk. Framingham Chart Determine your 10-year risk estimate for a heart attack. Use these charts to help you determine your risk. When you’re ready to calculate your risk, use the Step 7 chart to keep track of your scores. If your score indicates increased risk or if. Lung and Blood Institute’s Framingham Heart Study based on a.
For the primary prevention of cardiovascular disease, the Framingham Risk Score (FRS) is the most well-known risk prediction method. However, there are limited data regarding physicians' method of risk assessment and guideline adherence in clinical practice.
In the PARADIGM (Primary cARe AuDIt of Global risk Management) study (March 2009–10), 105 primary care physicians across Canada prospectively collected data for 3015 patients (mean age 56 years, 59% men) without known cardiovascular disease, diabetes, or lipid-lowering medications at baseline. For each patient, the treating physician determined their cardiovascular risk, and reported the risk stratification method and subsequent treatment decisions. Kappa statistics assessed the agreement between the study-calculated FRS and the treating physician's reported risk assessment. The FRS was the most commonly reported risk assessment method, but was used in only 34.0% of patients. Regardless of the method used (even if the FRS was reportedly used), there was only fair agreement between the risk stratification as reported by the physician and the study-calculated FRS. Moreover, physicians recommended statin initiation in 92% of all patients that they identified as high risk; however, according to the study-calculated FRS, only 56% of the truly high-risk patients were recommended statin therapy.
For the primary prevention of cardiovascular disease, these findings indicate a need to improve risk assessment and stratification, as misclassification directly contributes to suboptimal risk factor management in real-world clinical practice. Future studies should establish the optimal risk stratification method with quality improvement strategies for its subsequent implementation.
http://clinicaltrials.gov/ct2/show/NCT00950703; NCT00950703.
Introduction
In 2009, over 68 000 deaths in Canada were attributed to major cardiovascular diseases.1 While this remains high, appropriate risk assessment and risk factor management have resulted in a significant reduction of cardiovascular morbidity and mortality in Canada.2,3
Risk prediction tools4–7 can identify patients most likely to benefit from intervention, thereby guiding management decisions. Although the Framingham Risk Score (FRS) is the most well-known risk prediction method for the primary prevention of cardiovascular disease, other objective risk assessment options include the Reynold's Risk Score (RRS), high-sensitivity C-reactive protein, total number of risk factors, and carotid ultrasound to detect atherosclerosis.8 Alternately, physicians may use their overall subjective clinical assessment to estimate risk and guide management decisions.
Previous studies in acute coronary syndromes (ACSs) have described the discrepancies between objective and subjective global risk assessment.9–12 These discrepancies may contribute to important care gaps such as suboptimal treatment regimens and failure to reach guideline-recommended targets, and therefore suboptimal outcomes.9–12 Whether such discrepancies in risk assessment and guideline adherence exist in the primary prevention of cardiovascular disease in ‘real-world’ clinical practice is currently unknown.
In this observational study, we used data from the PARADIGM (Primary cAReAuDIt of Global risk Management) study to: (i) examine primary care physician assessment of cardiovascular risk in patients without known cardiovascular disease; (ii) evaluate the level of agreement of risk assessment between treating physicians and the FRS; and (iii) compare actual practice with established guidelines.
Methods
Setting
The PARADIGM study was a multicentre, prospective study that occurred between March 2009 and March 2010. It was approved by a central ethics review board (IRB Services). Primary care physicians whose practices included the management of patients for the primary prevention of cardiovascular disease (as assumed by their high statin prescribing patterns) were recruited to participate in the study.
Patients
From 10 provinces across Canada, 105 treating physician investigators consented and enrolled 3015 eligible patients into the study. Investigators prospectively collected and reported data using a standardized case report form. The inclusion criteria were asymptomatic, non-diabetic men (≥40 years), or women (≥50 years), who were seen in the office within the last year for a cardiovascular risk assessment. The exclusion criteria were history of diabetes or atherosclerosis (i.e. myocardial infarction, stroke, peripheral arterial disease, angina, or transient ischaemic attack), active liver disease or hepatic dysfunction (ALT >2 × upper limit of normal), active renal disease (baseline creatinine >170 µmol/L), history of malignancy within the past 5 years, chronic inflammatory conditions (i.e. arthritis, lupus, and inflammatory bowel disease), and known alcohol or drug abuse. Patients who had prior or current use of lipid-lowering therapies (statins, ezetimibe, fibrates, niacin, and fish oil), postmenopausal hormone replacement therapy, immunosuppressive medications, or oral steroids were also excluded. Patients who were known to have a high baseline cardiovascular risk (high risk by an FRS) were also excluded.
Main measurements
The case report form recorded data on demographics, medical history, medication use, and physical examination (such as anthropometric measurements and blood pressure). Laboratory investigations were performed within 6 weeks of the initial visit. At the second visit (<60 days after the initial visit), the treating physician re-assessed the patient and reported the patient's global cardiovascular risk as either low, intermediate, or high risk. Additionally, the physician recorded their choice of risk assessment method, and if applicable, the choice of drug therapy (for lipid or blood pressure management).
Study design
Continuous variables are presented as median (interquartile ranges) and compared by the Mann–Whitney U test. Categorical variables are presented as percentages and compared by the χ2 test. Guideline comparisons were made with the contemporary 2009 Canadian Cardiovascular Society (CCS)7 dyslipidemia guidelines, given that PARADIGM preceded the most recent 2012 CCS guidelines.6 Because the FRS is the most common risk stratification method and is also supported by the CCS, we used the recorded baseline data to formally calculate the 2008 FRS13 for a 10-year risk of cardiovascular disease (henceforth termed ‘study-calculated FRS’), as recommended in the 2009 CCS dyslipidemia guidelines. The study-calculated FRS was compared with the risk assessment reported by the treating physician. Kappa scores were used to assess the degree of agreement beyond chance: 0 (none), 0–0.2 (slight), 0.2–0.4 (fair), 0.4–0.6 (moderate), 0.6–0.8 (substantial), and 0.8–1.0 (almost perfect). Data analysis was performed using SPSS 21.0 (IBM), and P-values of <0.05 were considered to be statistically significant.
Results
In total, 3015 patients were enrolled in 2009–10. Table 1 summarizes their baseline characteristics, as stratified by the investigators' reported cardiovascular risk assessment of low (n = 1532), intermediate (n = 1093), or high (n = 390) risk. Patients reported to be at high risk were more likely to be male and older, with higher systolic blood pressures and LDL levels. At baseline, patients classified in the high-risk category were also more likely to be taking aspirin, angiotensin-converting enzyme inhibitors, calcium channel blockers, and diuretics.
Baseline patient characteristics
Overall (n = 3015)Risk-stratified by physician | P-value for trend | ||||
---|---|---|---|---|---|
Low risk (n = 1532) | Intermediate risk (n = 1093) | High risk (n = 390) | |||
Age (years)a | 56.3 | 54 (50–60) | 57 (52–63) | 58 (52–65) | |
Male, n (%) | 1774 | 811 (52.9%) | 701 (64.1%) | 262 (67.2%) | |
Ethnicity | |||||
European, n (%) | 2099 (69.6%) | 971 (46.3%) | 813 (38.7%) | 315 (15.0%) | <0.001 |
Chinese, n (%) | 266 (8.8%) | 194 (72.9%) | 61 (22.9%) | 11 (4.1%) | |
Other Asian, n (%) | 241 (8.0%) | 172 (71.4%) | 60 (24.9%) | 9 (3.7%) | |
South Asian, n (%) | 250 (8.3%) | 112 (44.8%) | 105 (42.0%) | 33 (13.2%) | |
Other, n (%) | 88 (2.9%) | 44 (50.0%) | 31 (35.2%) | 13 (14.8%) | |
African, n (%) | 71 (2.4%) | 39 (54.9%) | 23 (32.4%) | 9 (12.7%) | |
Co-morbidities | |||||
Hypertension, n (%) | 917 (30.4%) | 332 (21.7%) | 416 (38.1%) | 169 (43.3%) | <0.001 |
Treated hypertension, n (%) | 803 (26.6%) | 289 (18.9%) | 367 (33.6%) | 147 (37.7%) | <0.001 |
History of smoking, n (%) | 1046 (34.7%) | 410 (26.8%) | 429 (39.2%) | 207 (53.1%) | <0.001 |
First degree relative with vascular disease <60 years old, n (%) | 732 (24.3%) | 296 (19.4%) | 307 (28.1%) | 129 (33.2%) | <0.001 |
Health measurements | |||||
Systolic blood pressure (mmHg)a | 126.6 ± 14.0 | 122 (113–130) | 130 (120–137) | 130 (122–140) | <0.001 |
Diastolic blood pressure (mmHg)a | 78.5 ± 9.1 | 80 (70–82) | 80 (74–85) | 80 (73–86) | <0.001 |
Weight (kg)b | 79.2 ± 18.2 | 74.5 ± 17.0 | 82.7 ± 17.5 | 87.8 ± 19.0 | <0.001 |
Waist circumference (cm)b | 94.8 ± 13.6 | 91 ± 13 | 98 ± 12 | 102 ± 14 | <0.001 |
BMI (kg/m2)b | 27.81 ± 5.32 | 26.4 ± 4.6 | 28.7 ± 5.2 | 30.5 ± 5.7 | <0.001 |
LDL-C (mmol/L)b | 3.6 ± 0.8 | 3.40 ± 0.78 | 3.72 ± 0.82 | 3.80 ± 0.97 | <0.001 |
HDL-C (mmol/L)b | 1.4 ± 0.4 | 1.47 ± 0.41 | 1.32 ± 0.36 | 1.22 ± 0.34 | <0.001 |
Total cholesterol (mmol/L)b | 5.6 ± 1.0 | 5.46 ± 0.90 | 5.79 ± 0.98 | 5.93 ± 1.14 | <0.001 |
Triglyceride (mmol/L)b | 1.6 ± 1.0 | 1.34 ± 0.76 | 1.71 ± 1.16 | 2.06 ± 1.36 | <0.001 |
Fasting blood glucose (mmol/L)b | 5.4 ± 0.7 | 5.25 ± 0.62 | 5.43 ± 0.66 | 5.66 ± 1.11 | <0.001 |
HbA1c (%)b | 5.7 ± 0.5 | 5.7 ± 0.4 | 5.7 ± 0.4 | 5.7 ± 0.7 | <0.001 |
Creatinine (µmol/L)b | 80.0 ± 16.1 | 78 ± 15 | 82 ± 16 | 84 ± 17 | <0.001 |
eGFR (mL/min/1.73 m2)b | 77.4 ± 16.0 | 80.2 ± 16.1 | 75.4 ± 15.0 | 71.9 ± 16.0 | <0.001 |
hsCRP (mg/L)b | 2.6 ± 3.2 | 1.96 ± 3.17 | 3.45 ± 4.85 | 5.40 ± 4.86 | <0.001 |
Medication use at baseline visit | |||||
Aspirin | 406 (13.5%) | 139 (9.1%) | 194 (17.7%) | 73 (18.7%) | <0.001 |
ACE inhibitor | 327 (10.8%) | 115 (7.5%) | 151 (13.8%) | 61 (15.6%) | <0.001 |
Angiotension receptor blocker | 333 (11.0%) | 109 (7.1%) | 165 (15.1%) | 59 (15.1%) | <0.001 |
Beta-blocker | 120 (4.0%) | 49 (3.2%) | 49 (4.5%) | 22 (5.6%) | 0.014 |
Direct renin inhibitor | 13 (0.4%) | 2 (0.1%) | 6 (0.5%) | 5 (1.3%) | 0.002 |
Calcium channel blocker | 166 (5.5%) | 59 (3.9%) | 64 (5.9%) | 43 (11.0%) | <0.001 |
Diuretic | 444 (14.7%) | 148 (9.7%) | 200 (18.3%) | 96 (24.6%) | <0.001 |
Risk-stratified by physician | P-value for trend | ||||
---|---|---|---|---|---|
Low risk (n = 1532) | Intermediate risk (n = 1093) | High risk (n = 390) | |||
Age (years)a | 56.3 | 54 (50–60) | 57 (52–63) | 58 (52–65) | |
Male, n (%) | 1774 | 811 (52.9%) | 701 (64.1%) | 262 (67.2%) | |
Ethnicity | |||||
European, n (%) | 2099 (69.6%) | 971 (46.3%) | 813 (38.7%) | 315 (15.0%) | <0.001 |
Chinese, n (%) | 266 (8.8%) | 194 (72.9%) | 61 (22.9%) | 11 (4.1%) | |
Other Asian, n (%) | 241 (8.0%) | 172 (71.4%) | 60 (24.9%) | 9 (3.7%) | |
South Asian, n (%) | 250 (8.3%) | 112 (44.8%) | 105 (42.0%) | 33 (13.2%) | |
Other, n (%) | 88 (2.9%) | 44 (50.0%) | 31 (35.2%) | 13 (14.8%) | |
African, n (%) | 71 (2.4%) | 39 (54.9%) | 23 (32.4%) | 9 (12.7%) | |
Co-morbidities | |||||
Hypertension, n (%) | 917 (30.4%) | 332 (21.7%) | 416 (38.1%) | 169 (43.3%) | <0.001 |
Treated hypertension, n (%) | 803 (26.6%) | 289 (18.9%) | 367 (33.6%) | 147 (37.7%) | <0.001 |
History of smoking, n (%) | 1046 (34.7%) | 410 (26.8%) | 429 (39.2%) | 207 (53.1%) | <0.001 |
First degree relative with vascular disease <60 years old, n (%) | 732 (24.3%) | 296 (19.4%) | 307 (28.1%) | 129 (33.2%) | <0.001 |
Health measurements | |||||
Systolic blood pressure (mmHg)a | 126.6 ± 14.0 | 122 (113–130) | 130 (120–137) | 130 (122–140) | <0.001 |
Diastolic blood pressure (mmHg)a | 78.5 ± 9.1 | 80 (70–82) | 80 (74–85) | 80 (73–86) | <0.001 |
Weight (kg)b | 79.2 ± 18.2 | 74.5 ± 17.0 | 82.7 ± 17.5 | 87.8 ± 19.0 | <0.001 |
Waist circumference (cm)b | 94.8 ± 13.6 | 91 ± 13 | 98 ± 12 | 102 ± 14 | <0.001 |
BMI (kg/m2)b | 27.81 ± 5.32 | 26.4 ± 4.6 | 28.7 ± 5.2 | 30.5 ± 5.7 | <0.001 |
LDL-C (mmol/L)b | 3.6 ± 0.8 | 3.40 ± 0.78 | 3.72 ± 0.82 | 3.80 ± 0.97 | <0.001 |
HDL-C (mmol/L)b | 1.4 ± 0.4 | 1.47 ± 0.41 | 1.32 ± 0.36 | 1.22 ± 0.34 | <0.001 |
Total cholesterol (mmol/L)b | 5.6 ± 1.0 | 5.46 ± 0.90 | 5.79 ± 0.98 | 5.93 ± 1.14 | <0.001 |
Triglyceride (mmol/L)b | 1.6 ± 1.0 | 1.34 ± 0.76 | 1.71 ± 1.16 | 2.06 ± 1.36 | <0.001 |
Fasting blood glucose (mmol/L)b | 5.4 ± 0.7 | 5.25 ± 0.62 | 5.43 ± 0.66 | 5.66 ± 1.11 | <0.001 |
HbA1c (%)b | 5.7 ± 0.5 | 5.7 ± 0.4 | 5.7 ± 0.4 | 5.7 ± 0.7 | <0.001 |
Creatinine (µmol/L)b | 80.0 ± 16.1 | 78 ± 15 | 82 ± 16 | 84 ± 17 | <0.001 |
eGFR (mL/min/1.73 m2)b | 77.4 ± 16.0 | 80.2 ± 16.1 | 75.4 ± 15.0 | 71.9 ± 16.0 | <0.001 |
hsCRP (mg/L)b | 2.6 ± 3.2 | 1.96 ± 3.17 | 3.45 ± 4.85 | 5.40 ± 4.86 | <0.001 |
Medication use at baseline visit | |||||
Aspirin | 406 (13.5%) | 139 (9.1%) | 194 (17.7%) | 73 (18.7%) | <0.001 |
ACE inhibitor | 327 (10.8%) | 115 (7.5%) | 151 (13.8%) | 61 (15.6%) | <0.001 |
Angiotension receptor blocker | 333 (11.0%) | 109 (7.1%) | 165 (15.1%) | 59 (15.1%) | <0.001 |
Beta-blocker | 120 (4.0%) | 49 (3.2%) | 49 (4.5%) | 22 (5.6%) | 0.014 |
Direct renin inhibitor | 13 (0.4%) | 2 (0.1%) | 6 (0.5%) | 5 (1.3%) | 0.002 |
Calcium channel blocker | 166 (5.5%) | 59 (3.9%) | 64 (5.9%) | 43 (11.0%) | <0.001 |
Diuretic | 444 (14.7%) | 148 (9.7%) | 200 (18.3%) | 96 (24.6%) | <0.001 |
hsCRP, high-sensitivity C-reactive protein; eGFR, estimated glomerular filtration rate; ACE, angiotensin-converting enzyme.
Baseline patient characteristics
Overall (n = 3015)Risk-stratified by physician | P-value for trend | ||||
---|---|---|---|---|---|
Low risk (n = 1532) | Intermediate risk (n = 1093) | High risk (n = 390) | |||
Age (years)a | 56.3 | 54 (50–60) | 57 (52–63) | 58 (52–65) | |
Male, n (%) | 1774 | 811 (52.9%) | 701 (64.1%) | 262 (67.2%) | |
Ethnicity | |||||
European, n (%) | 2099 (69.6%) | 971 (46.3%) | 813 (38.7%) | 315 (15.0%) | <0.001 |
Chinese, n (%) | 266 (8.8%) | 194 (72.9%) | 61 (22.9%) | 11 (4.1%) | |
Other Asian, n (%) | 241 (8.0%) | 172 (71.4%) | 60 (24.9%) | 9 (3.7%) | |
South Asian, n (%) | 250 (8.3%) | 112 (44.8%) | 105 (42.0%) | 33 (13.2%) | |
Other, n (%) | 88 (2.9%) | 44 (50.0%) | 31 (35.2%) | 13 (14.8%) | |
African, n (%) | 71 (2.4%) | 39 (54.9%) | 23 (32.4%) | 9 (12.7%) | |
Co-morbidities | |||||
Hypertension, n (%) | 917 (30.4%) | 332 (21.7%) | 416 (38.1%) | 169 (43.3%) | <0.001 |
Treated hypertension, n (%) | 803 (26.6%) | 289 (18.9%) | 367 (33.6%) | 147 (37.7%) | <0.001 |
History of smoking, n (%) | 1046 (34.7%) | 410 (26.8%) | 429 (39.2%) | 207 (53.1%) | <0.001 |
First degree relative with vascular disease <60 years old, n (%) | 732 (24.3%) | 296 (19.4%) | 307 (28.1%) | 129 (33.2%) | <0.001 |
Health measurements | |||||
Systolic blood pressure (mmHg)a | 126.6 ± 14.0 | 122 (113–130) | 130 (120–137) | 130 (122–140) | <0.001 |
Diastolic blood pressure (mmHg)a | 78.5 ± 9.1 | 80 (70–82) | 80 (74–85) | 80 (73–86) | <0.001 |
Weight (kg)b | 79.2 ± 18.2 | 74.5 ± 17.0 | 82.7 ± 17.5 | 87.8 ± 19.0 | <0.001 |
Waist circumference (cm)b | 94.8 ± 13.6 | 91 ± 13 | 98 ± 12 | 102 ± 14 | <0.001 |
BMI (kg/m2)b | 27.81 ± 5.32 | 26.4 ± 4.6 | 28.7 ± 5.2 | 30.5 ± 5.7 | <0.001 |
LDL-C (mmol/L)b | 3.6 ± 0.8 | 3.40 ± 0.78 | 3.72 ± 0.82 | 3.80 ± 0.97 | <0.001 |
HDL-C (mmol/L)b | 1.4 ± 0.4 | 1.47 ± 0.41 | 1.32 ± 0.36 | 1.22 ± 0.34 | <0.001 |
Total cholesterol (mmol/L)b | 5.6 ± 1.0 | 5.46 ± 0.90 | 5.79 ± 0.98 | 5.93 ± 1.14 | <0.001 |
Triglyceride (mmol/L)b | 1.6 ± 1.0 | 1.34 ± 0.76 | 1.71 ± 1.16 | 2.06 ± 1.36 | <0.001 |
Fasting blood glucose (mmol/L)b | 5.4 ± 0.7 | 5.25 ± 0.62 | 5.43 ± 0.66 | 5.66 ± 1.11 | <0.001 |
HbA1c (%)b | 5.7 ± 0.5 | 5.7 ± 0.4 | 5.7 ± 0.4 | 5.7 ± 0.7 | <0.001 |
Creatinine (µmol/L)b | 80.0 ± 16.1 | 78 ± 15 | 82 ± 16 | 84 ± 17 | <0.001 |
eGFR (mL/min/1.73 m2)b | 77.4 ± 16.0 | 80.2 ± 16.1 | 75.4 ± 15.0 | 71.9 ± 16.0 | <0.001 |
hsCRP (mg/L)b | 2.6 ± 3.2 | 1.96 ± 3.17 | 3.45 ± 4.85 | 5.40 ± 4.86 | <0.001 |
Medication use at baseline visit | |||||
Aspirin | 406 (13.5%) | 139 (9.1%) | 194 (17.7%) | 73 (18.7%) | <0.001 |
ACE inhibitor | 327 (10.8%) | 115 (7.5%) | 151 (13.8%) | 61 (15.6%) | <0.001 |
Angiotension receptor blocker | 333 (11.0%) | 109 (7.1%) | 165 (15.1%) | 59 (15.1%) | <0.001 |
Beta-blocker | 120 (4.0%) | 49 (3.2%) | 49 (4.5%) | 22 (5.6%) | 0.014 |
Direct renin inhibitor | 13 (0.4%) | 2 (0.1%) | 6 (0.5%) | 5 (1.3%) | 0.002 |
Calcium channel blocker | 166 (5.5%) | 59 (3.9%) | 64 (5.9%) | 43 (11.0%) | <0.001 |
Diuretic | 444 (14.7%) | 148 (9.7%) | 200 (18.3%) | 96 (24.6%) | <0.001 |
Risk-stratified by physician | P-value for trend | ||||
---|---|---|---|---|---|
Low risk (n = 1532) | Intermediate risk (n = 1093) | High risk (n = 390) | |||
Age (years)a | 56.3 | 54 (50–60) | 57 (52–63) | 58 (52–65) | |
Male, n (%) | 1774 | 811 (52.9%) | 701 (64.1%) | 262 (67.2%) | |
Ethnicity | |||||
European, n (%) | 2099 (69.6%) | 971 (46.3%) | 813 (38.7%) | 315 (15.0%) | <0.001 |
Chinese, n (%) | 266 (8.8%) | 194 (72.9%) | 61 (22.9%) | 11 (4.1%) | |
Other Asian, n (%) | 241 (8.0%) | 172 (71.4%) | 60 (24.9%) | 9 (3.7%) | |
South Asian, n (%) | 250 (8.3%) | 112 (44.8%) | 105 (42.0%) | 33 (13.2%) | |
Other, n (%) | 88 (2.9%) | 44 (50.0%) | 31 (35.2%) | 13 (14.8%) | |
African, n (%) | 71 (2.4%) | 39 (54.9%) | 23 (32.4%) | 9 (12.7%) | |
Co-morbidities | |||||
Hypertension, n (%) | 917 (30.4%) | 332 (21.7%) | 416 (38.1%) | 169 (43.3%) | <0.001 |
Treated hypertension, n (%) | 803 (26.6%) | 289 (18.9%) | 367 (33.6%) | 147 (37.7%) | <0.001 |
History of smoking, n (%) | 1046 (34.7%) | 410 (26.8%) | 429 (39.2%) | 207 (53.1%) | <0.001 |
First degree relative with vascular disease <60 years old, n (%) | 732 (24.3%) | 296 (19.4%) | 307 (28.1%) | 129 (33.2%) | <0.001 |
Health measurements | |||||
Systolic blood pressure (mmHg)a | 126.6 ± 14.0 | 122 (113–130) | 130 (120–137) | 130 (122–140) | <0.001 |
Diastolic blood pressure (mmHg)a | 78.5 ± 9.1 | 80 (70–82) | 80 (74–85) | 80 (73–86) | <0.001 |
Weight (kg)b | 79.2 ± 18.2 | 74.5 ± 17.0 | 82.7 ± 17.5 | 87.8 ± 19.0 | <0.001 |
Waist circumference (cm)b | 94.8 ± 13.6 | 91 ± 13 | 98 ± 12 | 102 ± 14 | <0.001 |
BMI (kg/m2)b | 27.81 ± 5.32 | 26.4 ± 4.6 | 28.7 ± 5.2 | 30.5 ± 5.7 | <0.001 |
LDL-C (mmol/L)b | 3.6 ± 0.8 | 3.40 ± 0.78 | 3.72 ± 0.82 | 3.80 ± 0.97 | <0.001 |
HDL-C (mmol/L)b | 1.4 ± 0.4 | 1.47 ± 0.41 | 1.32 ± 0.36 | 1.22 ± 0.34 | <0.001 |
Total cholesterol (mmol/L)b | 5.6 ± 1.0 | 5.46 ± 0.90 | 5.79 ± 0.98 | 5.93 ± 1.14 | <0.001 |
Triglyceride (mmol/L)b | 1.6 ± 1.0 | 1.34 ± 0.76 | 1.71 ± 1.16 | 2.06 ± 1.36 | <0.001 |
Fasting blood glucose (mmol/L)b | 5.4 ± 0.7 | 5.25 ± 0.62 | 5.43 ± 0.66 | 5.66 ± 1.11 | <0.001 |
HbA1c (%)b | 5.7 ± 0.5 | 5.7 ± 0.4 | 5.7 ± 0.4 | 5.7 ± 0.7 | <0.001 |
Creatinine (µmol/L)b | 80.0 ± 16.1 | 78 ± 15 | 82 ± 16 | 84 ± 17 | <0.001 |
eGFR (mL/min/1.73 m2)b | 77.4 ± 16.0 | 80.2 ± 16.1 | 75.4 ± 15.0 | 71.9 ± 16.0 | <0.001 |
hsCRP (mg/L)b | 2.6 ± 3.2 | 1.96 ± 3.17 | 3.45 ± 4.85 | 5.40 ± 4.86 | <0.001 |
Medication use at baseline visit | |||||
Aspirin | 406 (13.5%) | 139 (9.1%) | 194 (17.7%) | 73 (18.7%) | <0.001 |
ACE inhibitor | 327 (10.8%) | 115 (7.5%) | 151 (13.8%) | 61 (15.6%) | <0.001 |
Angiotension receptor blocker | 333 (11.0%) | 109 (7.1%) | 165 (15.1%) | 59 (15.1%) | <0.001 |
Beta-blocker | 120 (4.0%) | 49 (3.2%) | 49 (4.5%) | 22 (5.6%) | 0.014 |
Direct renin inhibitor | 13 (0.4%) | 2 (0.1%) | 6 (0.5%) | 5 (1.3%) | 0.002 |
Calcium channel blocker | 166 (5.5%) | 59 (3.9%) | 64 (5.9%) | 43 (11.0%) | <0.001 |
Diuretic | 444 (14.7%) | 148 (9.7%) | 200 (18.3%) | 96 (24.6%) | <0.001 |
hsCRP, high-sensitivity C-reactive protein; eGFR, estimated glomerular filtration rate; ACE, angiotensin-converting enzyme.
In comparison with the physician-determined level of risk, as demonstrated in Table 2, the study-calculated FRS classified more patients as high risk (n = 679), whereas fewer patients were classified as low (n = 1314) or intermediate risk (n = 1021). Specifically, physicians identified 12.9% of all patients as high risk, whereas the study-calculated FRS classified 22.5% of all patients as high risk.
Physician-reported risk assessment vs. study-calculated FRS for all patients
Study-calculated FRS stratificationLow | Intermediate | High | Total | |
---|---|---|---|---|
Physician-reported risk stratification | ||||
Low | 947 | 446 | 138 | 1531 |
Intermediate | 299 | 452 | 342 | 1093 |
High | 68 | 123 | 199 | 390 |
Total | 1314 | 1021 | 679 | 3014 |
Low | Intermediate | High | Total | |
---|---|---|---|---|
Physician-reported risk stratification | ||||
Low | 947 | 446 | 138 | 1531 |
Intermediate | 299 | 452 | 342 | 1093 |
High | 68 | 123 | 199 | 390 |
Total | 1314 | 1021 | 679 | 3014 |
Physician-reported risk assessment vs. study-calculated FRS for all patients
Study-calculated FRS stratificationLow | Intermediate | High | Total | |
---|---|---|---|---|
Physician-reported risk stratification | ||||
Low | 947 | 446 | 138 | 1531 |
Intermediate | 299 | 452 | 342 | 1093 |
High | 68 | 123 | 199 | 390 |
Total | 1314 | 1021 | 679 | 3014 |
Low | Intermediate | High | Total | |
---|---|---|---|---|
Physician-reported risk stratification | ||||
Low | 947 | 446 | 138 | 1531 |
Intermediate | 299 | 452 | 342 | 1093 |
High | 68 | 123 | 199 | 390 |
Total | 1314 | 1021 | 679 | 3014 |
Figure 1 presents the method of cardiovascular risk assessment as reported by the treating physician. Approximately one-third of patients were stratified via the FRS, while subjective clinical judgement was used to stratify another third of patients, and alternative methods were applied for the remaining third.
Physician-reported risk assessment method (n = 3015).
Physician-reported risk assessment method (n = 3015).
In Tables 2–4, kappa scores are used to illustrate the level of agreement between the study-calculated FRS and the physician's estimate of cardiovascular risk. For all patients (n = 3014), the overall agreement between the study-calculated FRS and physician-estimated risk was only fair (kappa score 0.25). Similarly, when the treating physicians reported using a risk stratification method other than FRS (n = 1988), the kappa score was 0.22 (fair agreement). However, even when the treating physicians reported using the FRS (n = 1026), the kappa score was only 0.31 (fair agreement) when compared with the study-calculated FRS.
Physician-reported (non-FRS) vs. study-calculated FRS
Study-calculated FRS stratificationLow | Intermediate | High | Total | |
---|---|---|---|---|
Physician-reported risk stratification | ||||
Low | 570 | 250 | 86 | 906 |
Intermediate | 256 | 312 | 215 | 783 |
High | 66 | 103 | 130 | 299 |
Total | 892 | 665 | 431 | 1988 |
Low | Intermediate | High | Total | |
---|---|---|---|---|
Physician-reported risk stratification | ||||
Low | 570 | 250 | 86 | 906 |
Intermediate | 256 | 312 | 215 | 783 |
High | 66 | 103 | 130 | 299 |
Total | 892 | 665 | 431 | 1988 |
Physician-reported (non-FRS) vs. study-calculated FRS
Study-calculated FRS stratificationLow | Intermediate | High | Total | |
---|---|---|---|---|
Physician-reported risk stratification | ||||
Low | 570 | 250 | 86 | 906 |
Intermediate | 256 | 312 | 215 | 783 |
High | 66 | 103 | 130 | 299 |
Total | 892 | 665 | 431 | 1988 |
Low | Intermediate | High | Total | |
---|---|---|---|---|
Physician-reported risk stratification | ||||
Low | 570 | 250 | 86 | 906 |
Intermediate | 256 | 312 | 215 | 783 |
High | 66 | 103 | 130 | 299 |
Total | 892 | 665 | 431 | 1988 |
Physician-reported (FRS) vs. study-calculated FRS
Study-calculated FRS stratificationLow | Intermediate | High | Total | |
---|---|---|---|---|
Physician-reported risk stratification | ||||
Low | 377 | 196 | 52 | 625 |
Intermediate | 43 | 140 | 127 | 310 |
High | 2 | 20 | 69 | 91 |
Total | 422 | 356 | 248 | 1026 |
Low | Intermediate | High | Total | |
---|---|---|---|---|
Physician-reported risk stratification | ||||
Low | 377 | 196 | 52 | 625 |
Intermediate | 43 | 140 | 127 | 310 |
High | 2 | 20 | 69 | 91 |
Total | 422 | 356 | 248 | 1026 |
Physician-reported (FRS) vs. study-calculated FRS
Study-calculated FRS stratificationLow | Intermediate | High | Total | |
---|---|---|---|---|
Physician-reported risk stratification | ||||
Low | 377 | 196 | 52 | 625 |
Intermediate | 43 | 140 | 127 | 310 |
High | 2 | 20 | 69 | 91 |
Total | 422 | 356 | 248 | 1026 |
Low | Intermediate | High | Total | |
---|---|---|---|---|
Physician-reported risk stratification | ||||
Low | 377 | 196 | 52 | 625 |
Intermediate | 43 | 140 | 127 | 310 |
High | 2 | 20 | 69 | 91 |
Total | 422 | 356 | 248 | 1026 |
Table 5 and Figure 2 illustrate the percentage of patients in each risk category who were recommended statin therapy at the second visit, based on their risk assessment. Although statin initiation was recommended for 92% of all patients who were classified by their treating physicians as high risk, this was true for only 56% of the truly high-risk patients (as classified by the study-calculated FRS).
Statin initiation according to gender and risk stratification
Patient risk category, according to the treating physicianMen, n = 1771 | 4.7% | 47% | 93% | <0.001 |
Women, 1241 | 5.4% | 55% | 91% | <0.001 |
All, n = 3012 | 5.0% | 50% | 92% | <0.001 |
Patient risk category, based on the study-calculated FRS | ||||
Men, n = 1770 | 16% | 32% | 54% | <0.001 |
Women, n = 1241 | 20% | 41% | 68% | <0.001 |
All, n = 3011 | 18% | 35% | 56% | <0.001 |
Men, n = 1771 | 4.7% | 47% | 93% | <0.001 |
Women, 1241 | 5.4% | 55% | 91% | <0.001 |
All, n = 3012 | 5.0% | 50% | 92% | <0.001 |
Patient risk category, based on the study-calculated FRS | ||||
Men, n = 1770 | 16% | 32% | 54% | <0.001 |
Women, n = 1241 | 20% | 41% | 68% | <0.001 |
All, n = 3011 | 18% | 35% | 56% | <0.001 |
Statin initiation according to gender and risk stratification
Patient risk category, according to the treating physicianMen, n = 1771 | 4.7% | 47% | 93% | <0.001 |
Women, 1241 | 5.4% | 55% | 91% | <0.001 |
All, n = 3012 | 5.0% | 50% | 92% | <0.001 |
Patient risk category, based on the study-calculated FRS | ||||
Men, n = 1770 | 16% | 32% | 54% | <0.001 |
Women, n = 1241 | 20% | 41% | 68% | <0.001 |
All, n = 3011 | 18% | 35% | 56% | <0.001 |
Men, n = 1771 | 4.7% | 47% | 93% | <0.001 |
Women, 1241 | 5.4% | 55% | 91% | <0.001 |
All, n = 3012 | 5.0% | 50% | 92% | <0.001 |
Patient risk category, based on the study-calculated FRS | ||||
Men, n = 1770 | 16% | 32% | 54% | <0.001 |
Women, n = 1241 | 20% | 41% | 68% | <0.001 |
All, n = 3011 | 18% | 35% | 56% | <0.001 |
Statin initiation stratified according to risk for all patients (n = 3015).
Statin initiation stratified according to risk for all patients (n = 3015).
Conclusion
This multicentre, prospective study examined ‘real-world’ primary care practice patterns for primary prevention of cardiovascular disease. Primary care physicians reported that they were most likely to use the FRS for cardiovascular risk stratification. However, regardless of the method of risk assessment used by physicians, there was only fair agreement with the study-calculated FRS, even when physicians reported using the FRS. Although the physician-estimated cardiovascular risk appeared to appropriately guide lipid management through statin therapy, the initial misclassification of risk corresponded to suboptimal statin recommendations for patients at high risk for cardiovascular disease.
Depending on the method utilized, the resultant risk stratification may vary, which may lead to significant differences in subsequent management choices and outcomes. A large prospective case–cohort study directly compared several risk methods (FRS, Adult Treatment Panel III [ATP-III], and RRS), which resulted in risk scores ranging from 6 to 41% for a 10-year risk of ≥10% cardiovascular risk in a given population.14 However, specific patient characteristics, ease of use, pattern of practice, or data availability may guide the treating physician's choice of method. A US study demonstrated that although 92% of physicians were aware of risk stratification tools, they were only used in 41% of patients, with only a fraction of the latter being used to guide subsequent treatment decisions.15 A recent cross-sectional study surveyed 846 primary care physicians across Canada, and 68.9% of physicians reported using the FRS as their primary method of cardiovascular risk assessment.16 As our study demonstrates, even when physicians reported using the FRS, there was only fair agreement between the treating physicians' risk assessment and the study-calculated FRS. Although this raises concern about the actual usage or the ability to accurately apply the reported risk stratification method; given the fair agreement across all methods, perhaps in practice, the actual choice of method for risk stratification may not be as important as the mere use of any objective risk assessment method. In previous ACS studies, several objective risk assessment methods were compared with the physicians' subjective global risk assessment. The former were demonstrated to be superior in providing an additional prognostic value, with potentially improved management and outcomes.17
In comparison with the study-calculated FRS, the overall results suggest that physicians underestimated a significant proportion of patients with high risk, with consequent implications for management decisions. As demonstrated in Figure 2, when patients were identified as high risk by the treating physician, statins were recommended for over 90% of these patients, which corresponds to the CCS dyslipidemia guidelines.6,7 However, also illustrated by Figure 2, when these patients were re-assessed by the study-calculated FRS, statin therapy was actually recommended to just over half of patients who truly had a high cardiovascular risk. This finding is supported by previous data in the Canadian primary care physician population, which found that almost a third of the surveyed physicians were also unaware of the defining point for high risk (>20% 10-year FRS cardiovascular risk).16 This misunderstanding may have contributed to the misclassification of risk and underestimation of the truly high-risk patients, with a consequent effect on the subsequent lipid management decisions. This care gap is not unique to Canada, as similar findings exist in other countries; for example, a European study demonstrated that statins were only prescribed in 47% of patients with hypercholesterolaemia.18
The main strength of the study includes the PARADIGM study design, as it is a nation-wide registry with a large sample size. However, PARADIGM was not a population-based sampling of prescribers and patients, as physicians were recruited from a subset population of those with high statin prescribing patterns. Despite this limitation, these results likely represent more conservative findings, and true practice may be even more discordant with guidelines, which provides reason and an even greater impetus for improvement. Other study limitations include a clustering of responses, and the unrecorded rationale behind the physician's choice of cardiovascular risk assessment or management. Other limitations that were beyond the scope of the study include the lack of long-term data and outcomes, information regarding medication adherence and patient education, and any decisions that were deferred or subsequently changed after the second visit.
A need clearly exists to improve real-world cardiovascular risk stratification in primary cardiovascular disease prevention. Our findings suggest the need for appropriate use of validated risk assessment methods for appropriate risk factor management for the primary prevention of cardiovascular disease. The true adherence to lipid guidelines was suboptimal, at least in part due to the physician's misclassification of the patients' cardiovascular risk, which may contribute to suboptimal risk factor management. Further studies regarding the rationales for choosing methods or subsequent management decisions may be useful to identify quality improvement strategies. Moreover, consideration may be given towards the more widespread application of any validated objective risk assessment method, given the persisting fair agreement between the treating physician's reported risk assessment and the study-calculated FRS (regardless of the method reportedly used for risk stratification, including the FRS as the method reportedly used). Finally, future studies may need to establish the optimal risk stratification method, with subsequent quality improvement strategies to appropriately apply validated risk scores in primary prevention.
Funding
This study was funded by CCRN through an unrestricted investigator initiated research grant from AstraZeneca Canada, Inc.
Conflict of interest: none declared.
Acknowledgement
This research was conducted with support from the Investigator-Sponsored Study Programme of AstraZeneca.
References
;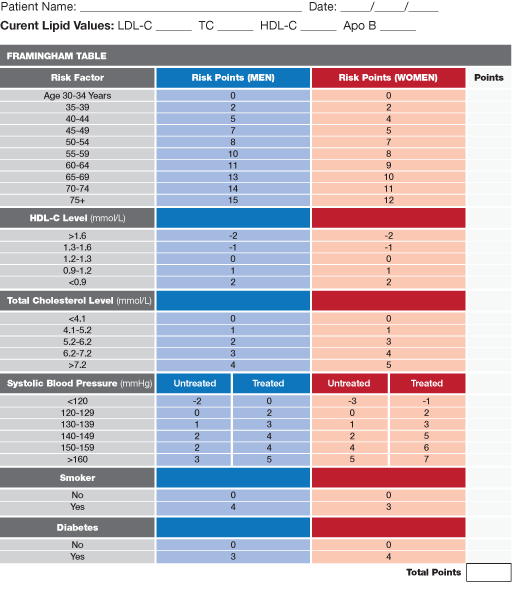

Supplementary data
Associated Data
Abstract
Background
Framingham-based and Reynolds risk scores for cardiovascular disease (CVD) prediction have not been directly compared in an independent validation cohort.
Methods and Results
We selected a case-cohort sample of the multi-ethnic Women’s Health Initiative Observational Cohort, comprising 1722 cases of major CVD (752 MIs, 754 ischemic strokes, and 216 other CVD deaths) and a random subcohort of 1994 women without prior CVD. We estimated risk using the ATP-III score, the Reynolds risk score, and the Framingham CVD model, reweighting to reflect cohort frequencies. Predicted 10-year risk varied widely between models, with 10% or higher risk in 6%, 10%, and 41% of women using the ATP-III, Reynolds, and Framingham CVD models, respectively. Calibration was adequate for the Reynolds model, but the ATP-III and Framingham CVD models over-estimated risk for CHD and major CVD, respectively. After recalibration, the Reynolds model demonstrated improved discrimination over the ATP-III model through a higher c-statistic (0.765 vs. 0.757, p=0.03), positive net reclassification improvement (NRI) (4.9%, p=0.02) and positive integrated discrimination improvement (IDI) (4.1%, p<0.0001) overall, excluding diabetics (NRI=4.2%, p=0.01), and in white (NRI=4.3%, p=0.04) and black (NRI=11.4, p=0.13) women. The Reynolds (NRI=12.9, p<0.0001) and ATP-III (NRI=5.9%, p=0.0001) models demonstrated better discrimination than the Framingham CVD model.
Conclusions
The Reynolds Risk Score was better calibrated than the Framingham-based models in this large external validation cohort. The Reynolds score also showed improved discrimination overall and in black and white women. Large differences in risk estimates exist between models, with clinical implications for statin therapy.
Introduction
Traditional Framingham risk factors of age, hypertension, smoking, diabetes, and total and HDL cholesterol form the basis for the Adult Treatment Panel III (ATP-III) coronary heart disease (CHD) risk prediction model. Cardiovascular risk, however, also relates to family history, markers of inflammation such as high-sensitivity C-reactive protein (hsCRP), and hemoglobin A1c (HbA1c) among diabetics. These additional biomarkers are included in the Reynolds Risk Score, an alternative global risk algorithm developed in 2007 for women and men.
Both the Framingham ATP-III and the Reynolds scores have received class I recommendations from the American College of Cardiology and the American Heart Association, and both scores are endorsed as part of the national guidelines for cardiovascular disease prevention in Canada. However, to date there has been no direct comparison of these two risk scoring systems in an independent prospective cohort that was not used in the derivation of either score. In addition, a Framingham prediction model has recently been developed for total cardiovascular disease (CVD), but this has not yet been validated in an external population.
All of these risk models for CVD have been developed primarily among white men and women, with little validation in multi-ethnic populations., A Framingham risk model for hard CHD events was validated in subcohorts of black and Native American women, but these included very small numbers of events. Other studies did not have the same success in validating a Framingham model in various populations., How well these models fit in diverse populations remains to be determined.
To address these issues, we directly examined the clinical performance of the Framingham and Reynolds scores in a case-cohort analysis conducted within the Women’s Health Initiative Observational Study (WHI-OS), a multi-ethnic, prospective cohort of more than 90,000 initially healthy postmenopausal American women. Specifically, we directly compared model fit in this independent validation cohort of women for three prediction algorithms: the Framingham score currently used in the ATP-III guidelines, the Reynolds Risk Score, and the Framingham score for total CVD. As all three scores were derived in predominantly white populations, the WHI-OS provided the opportunity to address their performance in a multi-ethnic population, and separately in black and white subgroups.
Methods
Women were participants in the WHI-OS and its long term follow-up, the WHI Extension Study. The WHI-OS includes 93,676 ethnically diverse postmenopausal women aged 50 to 79 years recruited between 1994 and 1998 at 40 clinical centers targeting minority groups to obtain a cross-section of the US population. Of these, 71,872 had no prior history of myocardial infarction (MI), stroke, revascularization procedures, pulmonary embolism, deep vein thrombosis, peripheral vascular disease, or cancer, and 60,890 additionally had baseline blood specimens and baseline risk factor information.
The WHI Clinical Coordinating Center collected baseline information on sociodemographic characteristics, lifestyle factors, health behaviors, and medical history, including blood pressure measurements. Diabetes and family history, defined here as MI before age 55 in men and 65 in women, were self-reported. Participants brought current medications to clinic visits to assess medication use.
Self-reported outcome data through September 2008 were confirmed through medical record review by centrally trained physicians. MI and coronary death were combined for the CHD outcome. Medical records, electrocardiogram readings, and cardiac enzyme and troponin determinations were used for confirmation. Strokes were defined as rapid onset of a persistent neurologic deficit attributed to an obstruction or rupture of the brain arterial system, lasting more than 24 hours and without evidence of other cause. These were classified as ischemic or hemorrhagic through review of brain imaging study reports. Underlying cause of death was classified on the basis of death certificates, medical records, and other records such as autopsy reports. The primary endpoint for this analysis is a combined endpoint of major CVD, including CHD, ischemic stroke, and death due to cardiovascular causes. This project has been approved by the Institutional Review Board at the Brigham and Women’s Hospital, Boston, MA.
Sample selection
Because of the large size of the WHI, to reduce costs of biochemical assays a prospective case-cohort design14 was employed in this WHI substudy. To maximize efficiency for examining non-whites, selected cases included all eligible CVD cases from black (n=200), Hispanic (n=53), and Asian (n=55) women, and women with other/unknown ethnicity (n=55). For efficiency, the remaining 1637 of 2000 cases were randomly selected from 2370 cases among white women.
A subcohort of approximately 2000 women was selected using the same eligibility criteria and stratified to match cases by race/ethnicity and five-year age groups. Further exclusion for this analysis of those with other prior CVD conditions, including transient ischemic attack (TIA), CVD surgery, or congestive heart failure (CHF) led to a final sample size of 1,722 cases and a subcohort of size 1,994, of whom 121 were also cases. Among those in the subcohort who did not develop CVD, the median (25%, 75%) follow-up time was 9.9 (8.6, 11.8) years.
For women in the selected samples, blood specimens collected and stored at study entry were assayed centrally for total cholesterol, HDL cholesterol, hsCRP, and HbA1c (among diabetics) using standardized procedures. The core laboratory is certified by the National Heart, Lung, and Blood Institute/Centers for Disease Control and Prevention Lipid Standardization Program.
Statistical methods
The data were analyzed throughout as a case-cohort study14, using appropriate weighting of the observations. Because the numbers in the full sample were known, our stratified sampling enabled us to mimic or recapture the characteristics of the full WHI cohort using reweighting by the sampling frequency. Overall population characteristics were estimated using inverse probability weights in Proc Survey means in SAS 9.2.17 To first verify the associations of risk factors within the WHI sample, weighted Cox regression was used to estimate hazard ratios using Proc Phreg of SAS, and asymptotic variance estimates were computed following Langholz and Jiao.19 Continuous risk factors were treated in a continuous fashion as well as in clinical risk categories.
Predicted values for CHD and CVD were obtained using published equations from the Framingham risk scores for CHD and CVD and from the Reynolds models for CVD. Framingham risk factors include age, blood pressure, antihypertensive treatment, smoking, diabetes, and total and HDL cholesterol. The ATP-III model is intended for those without diabetes, which is considered a risk equivalent. The Reynolds Risk Score additionally includes hsCRP, family history of premature MI (before age 60), and hemoglobin A1c among diabetics only. The fit of the models in the WHI data was examined using appropriate weighting. The c-statistic for survival data, was computed and differences between models assessed using bootstrapping. Calibration plots were used to compare observed and predicted risk within deciles using inverse sampling weights. In addition, for completeness, we also considered the alternative Framingham simple model which uses body mass index (BMI) instead of lipids (available at http://www.framinghamheartstudy.org/risk/gencardio.html#).
Because different endpoint definitions were used in development of each of the three models, recalibration was necessary to compare models using reclassification methods. The Framingham ATP-III score predicts ‘hard’ CHD, defined as MI and coronary death, while the Reynolds Risk Score predicts a composite CVD outcome defined as incident MI, ischemic stroke, coronary revascularization, and cardiovascular death. The Framingham CVD score predicts ‘total’ CVD defined as all coronary events (MI, coronary death, coronary insufficiency, and angina), cerebrovascular events (including ischemic stroke, hemorrhagic stroke, and TIA), peripheral artery disease (intermittent claudication), and heart failure. As described above, the major CVD endpoint used in these WHI data comprised CHD, ischemic stroke, and CVD death, and did not match any of these precisely. To correct for differences in endpoint definition, after initial evaluation of fit, models were recalibrated to the WHI cohort using logistic regression calibration for 10-year risk., This process does not change the coefficients for risk factors, but changes the intercept only, to alter the mean predicted risk. The average predicted risk for each model then approximately equaled the overall incidence of major CVD at ten years in the WHI cohort of eligible women.
We used plots to examine the calibration of original and recalibrated models. These plot the average predicted risk within deciles against the observed risk in that decile, adding a reference line for perfect calibration. To directly compare recalibrated models, we examined the integrated discrimination improvement (IDI) and reclassification statistics, including reclassification calibration chi-squares, and net reclassification improvement (NRI). For the reclassification tables, clinical-based cut points of 5, 10, and 20% were used. Survival methods were used throughout,, and measures were reweighted to reflect the distribution in the overall cohort. Statistical tests of reweighted measures were derived using bootstrap samples. Models were also examined among women without diabetes, eliminating those on statins or other cholesterol-lowering medications (7%), and separately among white and black women.
Results
Of 1,722 incident cardiovascular cases, 752 were MIs, 754 ischemic strokes, and 216 CVD deaths. Baseline characteristics for cases and the subcohort are shown in Table 1 both in the selected subsample and reweighted to the population distribution. In the subcohort sample the average age was 69 years with 5% current smokers and 5% diabetics. Reweighted population estimates were age 62 years, 6% current smokers, and 4% diabetics. Cases included more smokers and diabetics, and generally higher risk factor levels. Reweighted distributions in the subcohort are shown by race/ethnicity in Supplemental Table 1. Blacks were slightly younger than whites, but had higher proportions of smokers and diabetics.
Table 1
Baseline characteristics in ischemic CVD cases and subcohort members, among those with no prior CVD (including TIA, CHF, CVD surgery), crude and weighted to the population distribution.*
Risk Factor | Subcohort | All Cases | MI | Stroke | CVD Death |
---|---|---|---|---|---|
N | 1994 | 1722 | 752 | 754 | 216 |
Crude | |||||
Age (yrs) | 69 (63, 73) | 69 (64, 73) | 68 (63, 73) | 69 (64, 73) | 70 (64, 74) |
Current Smoking (%) | 4.9 | 8.9 | 9.0 | 7.8 | 12.0 |
Diabetes (%) | 4.7 | 10.7 | 13.0 | 9.4 | 7.4 |
HbA1c among diabetics (%) | 7.0 (6.2, 7.9) | 7.5 (6.8, 8.9) | 7.4 (6.5, 8.6) | 7.7 (6.8, 9.6) | 7.5 (7.0, 8.4) |
Systolic blood pressure (mmHg) | 128.0 (117, 140) | 134 (122, 148) | 133 (121, 147) | 135 (123, 149) | 133 (120, 142) |
Hypertension medication (%) | 26.6 | 38.3 | 38.3 | 38.6 | 37.0 |
Total Cholesterol (mg/dl) | 225 (200, 256) | 226 (198, 253) | 229 (202, 258) | 221 (196, 248) | 227 (202, 252) |
HDL Cholesterol (mg/dl) | 54.4 (44.6, 66.6) | 48.6 (39.8, 59.8) | 48.8 (39.8, 60.1) | 47.9 (38.8, 58.6) | 50.5 (42.3, 62.0) |
C-reactive protein (mg/L) | 2.3 (1.0, 5.0) | 3.1 (1.4, 6.2) | 3.2 (1.4, 6.2) | 2.9 (1.3,6.3) | 3.1 (1.5, 6.0) |
Family history of MI before age 65 (%) | 17.6 | 22.5 | 25.1 | 20.8 | 19.4 |
Weighted by Sample Frequencies | |||||
Age (yrs) | 62.0 (56.2, 67.8) | 68.2 (63.2, 72.6) | 67.8 (62.4, 72.3) | 68.3 (63.7, 72.6) | 69.2 (64.0, 73.6) |
Current Smoking (%) | 5.7 | 8.5 | 8.6 | 7.4 | 11.7 |
Diabetes (%) | 3.7 | 10.1 | 12.2 | 8.8 | 7.0 |
HbA1c if diabetic (%) | 7.0 (6.2, 8.0) | 7.5 (6.7, 8.8 | 7.4 (6.5, 8.6) | 7.6 (6.8, 9.2) | 7.3 (7.0, 8.4) |
Systolic blood pressure (mmHg) | 123.8 (113.9, 136.0) | 133.1 (121.5, 146.9) | 132.5 (120.6, 146.6) | 134.2 (122.6, 148.4) | 132.3 (120.4, 142.0) |
Hypertension medication (%) | 22.5 | 37.8 | 37.8 | 38.0 | 36.9 |
Total Cholesterol (mg/dl) | 223.8 (199.5, 255.7) | 225.2 (198.8, 252.7) | 228.6 (202.0, 259.1) | 220.2 (195.8, 247.5) | 227.0 (202.1, 252.9) |
HDL Cholesterol (mg/dl) | 54.5 (44.6, 66.5) | 48.7 (39.8, 59.8) | 48.9 (39.7, 60.1) | 48.0 (39.0, 58.5) | 50.4 (42.1, 62.2) |
C-reactive protein (mg/L) | 2.4 (1.0, 5.2) | 3.1 (1.4, 6.1) | 3.1 (1.4, 6.1) | 2.9 (1.3, 6.2) | 3.0 (1.4, 5.8) |
Family history of MI before age 65 (%) | 19.6 | 23.1 | 25.6 | 21.5 | 20.0 |
Risk factor associations
Multivariable Cox regression models confirmed the association of risk factors with CVD, both overall and among whites and blacks (Table 2). Each risk factor had significant associations in the overall sample except for total cholesterol, which was the same after excluding women on cholesterol-lowering medications. When examined separately using CHD as an endpoint, total cholesterol was a significant predictor (hazard ratio (HR) for total cholesterol ≥ 240 = 1.30, 95% confidence interval (CI) = 1.01–1.66). Estimated effects of each risk factor were generally consistent for whites and blacks, although results were more variable among blacks due to smaller numbers. The effect of measured blood pressure was weaker but anti-hypertensive medication stronger among blacks, and the effect of total cholesterol was stronger among black women. Age, diabetes, smoking, HDL-cholesterol, hsCRP, and family history all had significant independent effects on major CVD. Regressions using continuous versions of the risk factors showed similar results (Supplemental Table 2). Interactions of age and total cholesterol and of smoking and age included in the ATP-III model were not significant in these data, and are not included in these models.
Table 2
Results of multivariable Cox regression analysis, including all variables in the model, in case-cohort sample in ischemic CVD cases and subcohort members with no prior CVD – effects of risk factor categories.
Risk factor | Overall | Whites | Blacks |
---|---|---|---|
HR (95% CI) | HR (95% CI) | HR (95% CI) | |
Age (yrs) | |||
60–69 | 3.05 (2.53–3.69) | 3.39 (2.72–4.21) | 1.58 (0.92–2.71) |
70+ | 6.66 (5.48–8.09) | 7.12 (5.70–8.90) | 7.27 (3.99–13.25) |
Diabetes | 1.94 (1.40–2.67) | 1.52 (1.03–2.23) | 3.57 (1.75–7.31) |
Current smoking | 2.39 (1.76–3.26) | 2.06 (1.43–2.95) | 3.65 (1.63–8.18) |
SBP (mmHg) | |||
120–<140 | 1.38 (1.15–1.65) | 1.47 (1.21–1.79) | 0.67 (0.35–1.30) |
>=140 | 2.02 (1.65–2.49) | 2.10 (1.67–2.62) | 1.09 (0.54–2.20) |
Hypertension medication | 1.50 (1.26–1.77) | 1.49 (1.23–1.79) | 1.80 (1.08–3.01) |
Total cholesterol (mg/dl) | |||
200–<240 | 0.93 (0.76–1.13) | 0.93 (0.75–1.15) | 1.50 (0.75–3.00) |
>=240 | 1.06 (0.87–1.30) | 1.00 (0.81–1.25) | 2.18 (1.08–4.41) |
HDL cholesterol (mg/dl) | |||
40–<60 | 0.66 (0.54–0.82) | 0.66 (0.53–0.83) | 0.34 (0.17–0.71) |
>=60 | 0.42 (0.34–0.53) | 0.42 (0.33–0.54) | 0.32 (0.14–0.72) |
C-reactive protein (mg/L) | |||
1–<3 | 1.22 (0.99–1.50) | 1.21 (0.97–1.51) | 1.83 (0.79–4.22) |
>=3 | 1.45 (1.19–1.77) | 1.43 (1.15–1.78) | 1.75 (0.81–3.78) |
Family history of premature MI | 1.25 (1.03–1.52) | 1.25 (1.02–1.53) | 1.47 (0.70–3.07) |
Predicted risk and model fit
Predicted 10-year risk was estimated using published equations for each model. These varied widely between models, as shown in the distributions in Figure 1A. Average risk was 3.8%, 4.6%, and 10.9% for the ATP-III, Reynolds, and Framingham CVD models. Estimated risk was 10% or higher in 5.5%, 10.3%, and 41.1% of women, respectively (Figure 1B), and risk was 20% or higher in 0.5, 2.6, and 10.6% of women in the three models.
Distribution of risk estimated from the Framingham ATP-III score, the Reynolds risk score, and the Framingham CVD score among women in the WHI (A), and the estimated percent of women with risk of 10% or greater and 20% or greater using the published scores (B).
To assess calibration, predicted risk for the ATP-III score was compared to observed 10-year rates of CHD among non-diabetics only, to match its intended use (Figure 2A). As shown, the ATP-III model over-estimated risk of CHD, with predicted values higher than those observed. In contrast, the CHD score was actually better calibrated to the risk of major CVD among all women (Figure 2B). The Reynolds risk score appeared relatively well-calibrated for the endpoint of major CVD (Figure 2C). The Framingham CVD model, developed for a broader definition of CVD, greatly over-estimated risk of major CVD as anticipated (Figure 2D). Similar patterns of over-estimation of risk were seen for the ATP III model for CHD and the Framingham model for CVD among blacks (Figure 3) and whites (Supplemental Figure 1) separately, as the predicted risk was higher than the observed risk in each group.
Calibration plots for the original published risk prediction scores, including the ATP-III score and the coronary heart disease (CHD) outcome among only those without diabetes (A), and the ATP-III score and the cardiovascular disease (CVD) outcome (B), the Reynolds risk score and CVD (C), and the Framingham CVD score and CVD (D) among all women.
Calibration plots for the original published risk prediction scores among black women only, including the ATP-III score and the coronary heart disease (CHD) outcome among those without diabetes (A), and the ATP-III score and the cardiovascular disease (CVD) outcome (B), the Reynolds risk score and CVD (C), and the Framingham CVD score and CVD (D) among all black women.
In order to directly compare discrimination of the three models for the endpoint of CVD, the models were recalibrated such that the average predicted risk equaled the overall reweighted population estimate of 4%. The percent of women with estimated risk of 10% or higher was then 6.6%, 7.7%, and 6.2% for the ATP-III, Reynolds, and Framingham CVD model. After recalibration of the mean, all three models showed reasonable calibration to the major CVD endpoint (Supplemental Figure 2). C-statistics for major CVD (unaffected by recalibration) were 0.757 for the ATP-III model, 0.765 for the Reynolds model, and 0.750 for the Framingham CVD model. Differences in the c-statistics, though small, were all statistically significant (Table 3). When the simple non-lab based Framingham CVD model was considered, the calibration was very similar to that of the lab-based model. However, the discrimination was worse, with a c-statistic of 0.747, which was significantly lower than that for the Reynolds model (p=0.0004), but more similar to the lab-based Framingham CVD model (p=0.57) and the ATP-III model (P=0.055).
Table 3
Comparison of recalibrated models for CVD events in the WHI in ischemic CVD cases and subcohort members with no prior CVD, based on weighted survival estimates for case-cohort studies.
Model Comparison | Change in C-index | RC X2old* | RC X2new* | NRI (%) | Contin NRI (%) | IDI (%) |
---|---|---|---|---|---|---|
All Women | ||||||
RRS vs. ATP-III | 0.008 | 273.5 | 185.7 | 4.9 | 32.1 | 4.1 |
(p) | 0.032 | 0.010 | <0.0001 | <0.0001 | ||
Framingham CVD vs. ATP-III | −0.008 | 85.2 | 246.8 | −5.9 | −51.5 | 0.2 |
(p) | 0.020 | 0.0002 | <0.0001 | 0.42 | ||
RRS vs. Framingham CVD | 0.016 | 283.5 | 153.2 | 12.9 | 60.0 | 3.9 |
(p) | <0.0001 | <0.0001 | <0.0001 | <0.0001 | ||
Whites | ||||||
RRS vs. ATP-III | 0.007 | 132.0 | 198.8 | 4.3 | 30.8 | 4.3 |
(p) | 0.068 | 0.043 | <0.0001 | <0.0001 | ||
Framingham CVD vs. ATP-III | −0.008 | 80.2 | 227.1 | −6.9 | −52.8 | 0.3 |
(p) | 0.015 | <0.0001 | <0.0001 | 0.34 | ||
RRS vs. Framingham CVD | 0.016 | 275.6 | 186.4 | 13.0 | 58.6 | 4.1 |
(p) | <0.0001 | <0.0001 | <0.0001 | <0.0001 | ||
Blacks | ||||||
RRS vs. ATP-III | 0.007 | 15.2 | 8.0 | 11.4 | 32.9 | 3.5 |
(p) | 0.56 | 0.13 | 0.004 | 0.010 | ||
Framingham CVD vs. ATP-III | −0.013 | 6.8 | 15.7 | −2.1 | −30.1 | −0.1 |
(p) | 0.16 | 0.76 | 0.001 | 0.80 | ||
RRS vs. Framingham CVD | 0.020 | 41.2 | 14.0 | 23.7 | 76.7 | 3.6 |
(p) | 0.018 | 0.004 | <0.0001 | 0.0003 | ||
All Non-Diabetics | ||||||
RRS vs. ATP-III | 0.0008 | 114.2 | 103.5 | 4.2 | 28.1 | 1.5 |
(p) | 0.82 | 0.010 | <0.0001 | <0.0001 | ||
Framingham CVD vs. ATP-III | −0.016 | 70.3 | 225.8 | −9.0 | −61.4 | −0.6 |
(p) | <0.0001 | <0.0001 | <0.0001 | <0.0001 | ||
RRS vs. Framingham CVD | 0.016 | 246.0 | 39.0 | 13.4 | 59.7 | 2.2 |
(p) | <0.0001 | <0.0001 | <0.0001 | <0.0001 |
Risk reclassification
Fit of the recalibrated models was directly compared using reclassification measures (Table 3). Reclassification tables comparing the Reynolds to both Framingham models using both the crude and recalibrated predicted values are shown in Supplemental Tables 3A–D. These show the numbers of women in the total WHI cohort who would be reclassified into the various risk groups. For example, using the original scores in Table 3A, 664 (23%) of the 2,943 women at ATP III risk of 10–<20% would be reclassified as >=20% risk and 544 (18%) as less than 10% risk. In addition, of the 10,620 women originally at 5–<10% risk, 576 (5%) would be reclassified above 20% and 3,028 (29%) above 10%. Compared to the recalibrated ATP-III model, the Reynolds model showed improvement in discrimination based on the NRI (4.9%, 95%CI=1.2–8.7%, p=0.010), with improvement of 4.0% (p=0.02) in cases, and 1.0% (p=0.30) in non-cases. Improvement was also seen using the IDI (4.1%, 95%CI=2.7–5.7%, p<0.0001; relative IDI=117.3%), the continuous NRI (32.1%, 95%CI=24.2–39.5%, p<0.0001), and reclassification calibration chi-squares (273.5 vs. 185.7), indicating that calibration improved with the Reynolds model. When comparing the Framingham CVD model to the ATP-III model, the former provided a worse fit even though it was developed for the endpoint of CVD rather than CHD. The NRI and IDI were negative, calibration worse, and c-statistic lower (Table 3). Comparison of the Reynolds to the Framingham CVD model indicated improved fit on all measures, with an NRI of 12.9% (95%CI=9.4–16.3%, p<0.0001). Comparison of the Reynolds model to the simple non-lab based Framingham model was very similar with an NRI of 11.9% (p<0.0001).
Model fit statistics for recalibrated models were similar among whites only (Table 3). Although not always significant due to smaller numbers, the direction of effects was the same and often stronger among black women, with the Reynolds model showing improved fit over the ATP-III score, and both providing better fit than the Framingham CVD score.
Since the ATP-III model was intended for those without diabetes, we also recalibrated the models separately including only women without diabetes at baseline (Table 3). Although the c-statistics and reclassification calibration chi-squares were similar for the ATP-III and Reynolds models, the NRI showed a significant improvement favoring the Reynolds model (4.2%, 95%CI=1.1–7.5%, p=0.01), as did the continuous NRI and the IDI (both p<0.0001). Finally, results were very similar after excluding women on cholesterol-lowering medications (Supplemental Table 4).
Discussion
This validation study prospectively examined the fit of three cardiovascular risk prediction models in a large-scale external cohort, the WHI-OS, a national, geographically representative, racially and ethnically diverse sample of US women. Models included the Framingham-based ATP-III model, the Reynolds Risk Score, and the Framingham CVD model. The published ATP-III model was poorly calibrated for CHD, and the Reynolds Risk Score was better calibrated for major CVD than the Framingham CVD model. Moreover, after recalibration, the Reynolds Risk Score continued to show statistically significant but modest improvement in fit when compared to either of the Framingham-based models.
We know of no prior work directly comparing the Framingham and Reynolds scores in an independent prospective cohort. However, the findings here that family history, inflammation, and HbA1c among diabetics improve global risk prediction are consistent with observations made in other settings considering each factor in isolation.– Further, the lack of effect modification in our data by ethnicity suggests that these findings are clinically relevant in ethnically diverse populations.
Care must be used when interpreting these data, as the endpoints used to generate the three scores differ from each other and from the primary endpoint of the WHI-OS, which could lead to lack of calibration. However, the ATP-III score over-estimated risk of its intended endpoint, ‘hard CHD’ among non-diabetics, a finding replicated in the Women’s Health Study (data available on request). The WHI-OS sample is older than that of Framingham, and traditional risk markers are generally less predictive in older age ranges,, although the ATP-III score includes interactions of age with smoking and total cholesterol. One Framingham model has previously been validated in six prospective, ethnically diverse cohorts and was shown to have reasonable calibration overall, but prior data specifically for the ATP-III model are sparse. Other, primarily European, investigators have also reported that various versions of Framingham models over-predict CHD incidence,, Since clinicians in practice cannot re-calibrate a score for an individual patient, population calibration is essential.
The Framingham CVD model was also poorly calibrated for the endpoint of major CVD. However, it was developed for the broader endpoint of total CVD including several other conditions, namely angina, coronary insufficiency, TIA, peripheral artery disease, and CHF. As statins reduce stroke as well as CHD, scoring systems including stroke have recently been favored, whereas the inclusion of other endpoints such as CHF are more controversial. In this research setting, re-calibration allowed us to minimize differences in outcomes and directly compare risk scores for a comparable endpoint. As shown in the re-calibrated analyses, the Framingham CVD score, developed using an endpoint closer to that used here, was not superior to the ATP-III score for CHD only, and both of these discriminated less well than the Reynolds score. It is possible that the lower levels of discrimination seen with the Framingham CVD model may reflect reduced effects of the traditional risk factors on these secondary endpoints.
More importantly, the number of women potentially eligible for statin therapy can vary greatly depending on the equation and endpoint used. As shown in Figure 1, the estimated distributions of predicted risks in the population vary widely across models. Using the WHI data, in a hypothetical population of 100,000 women the number who would be classified at 10% or higher risk would be 5,549 with the published ATP-III model, 10,304 with the Reynolds score, and 41,074 with the Framingham CVD model (Supplemental Tables 3AC). Even if all models were perfectly calibrated for their respective outcomes, the choice of endpoints needs to be addressed. Whether statins are equally effective for the various CVD endpoints, and whether the risk-benefit equation is the same for all endpoints, are important criteria in developing guidelines for therapy.
We believe these data may have clinical implications for CVD prevention in otherwise healthy middle-aged women. Risk prediction algorithms have been widely used to better target cardiovascular preventive therapies, in particular the use of statins. A recent meta-analysis including 13,154 women in primary prevention statin trials reported a 37% reduction in cardiovascular event rates. However, the great majority of women destined to suffer a cardiovascular event have ATP-III scores less than 10 percent. These women would not qualify for treatment under current guidelines yet could benefit from statin therapy. Using data from the WHI-OS, among women with 10-year ATP-III risks of 5 to 10 percent, the Reynolds score would reclassify 15% to a lower risk category (< 5%) and over 28% to a higher risk category (> 10%), including 5% with estimated risk exceeding 20% (Supplemental Table 3A).
Limitations of our analysis merit consideration. First, the WHI-OS is composed exclusively of women, so these results cannot be generalized to men. However, prior work in the Physicians Health Study has shown that the Reynolds Risk Score for men improves fit and reclassification in that setting. Second, there may be remaining differences in endpoint definition. Confirmation procedures for Framingham, WHS, and the WHI-OS may have differed somewhat even for common endpoints such as CHD. Third, we were not able to fully assess the calibration of the Framingham CVD model since we did not have data available on the broader definition used for that model. Fourth, estimates from this case-cohort sample may offer less precision than those based on the full WHI cohort.
In sum, in this large scale comparison of risk prediction models commonly used in North America, the Reynolds Risk Score significantly improved fit as compared to either the Framingham-based ATP-III CHD risk score or the newer Framingham CVD score. Within the WHI-OS, the greatest impact of the Reynolds Risk Score appeared to be among those women with 5 to 10 percent 10-year estimated risk according to ATP-III, a group including a large number of women destined to suffer MI or stroke, and in whom trial data indicate efficacy of statin therapy in reducing cardiovascular events.
Supplementary Material
1
Acknowledgments
Women’s Health Initiative Investigators: A full listing of Women’s Health Initiative investigators can be found at http://whiscience.org/publications/WHI_investigators_longlist. Additional Contributions: We thank the Women’s Health Initiative investigators, staff, and study participants for their outstanding dedication and commitment. We also thank Dr. Bryan Langholz for advice concerning inference for the case-cohort sample.
Funding Sources: This project was supported by National Heart, Lung, and Blood Institute’s Broad Agency Announcement contract number HHSN268200960011C. The Women’s Health Initiative program is funded by the National Heart, Lung, and Blood Institute, the National Institutes of Health, and the US Department of Health and Human Services through contracts N01WH22110, 24152, 32100-2, 32105-6, 32108-9, 32111-13, 32115, 32118-9, 32122, 42107-26, 42129-32, and 44221.
Footnotes
Conflict of Interest Disclosures: Dr. Ridker is listed as a co-inventor on patents held by the Brigham and Women’s Hospital, Boston, MA, that relate to the use of inflammatory biomarkers in cardiovascular disease that have been licensed to AstraZeneca and Siemens. Drs. Ridker and Manson are listed as co-inventors on a patent held by Brigham and Women’s Hospital, Boston, MA, that relates to the use of inflammatory biomarkers in diabetes that has been licensed to AstraZeneca.